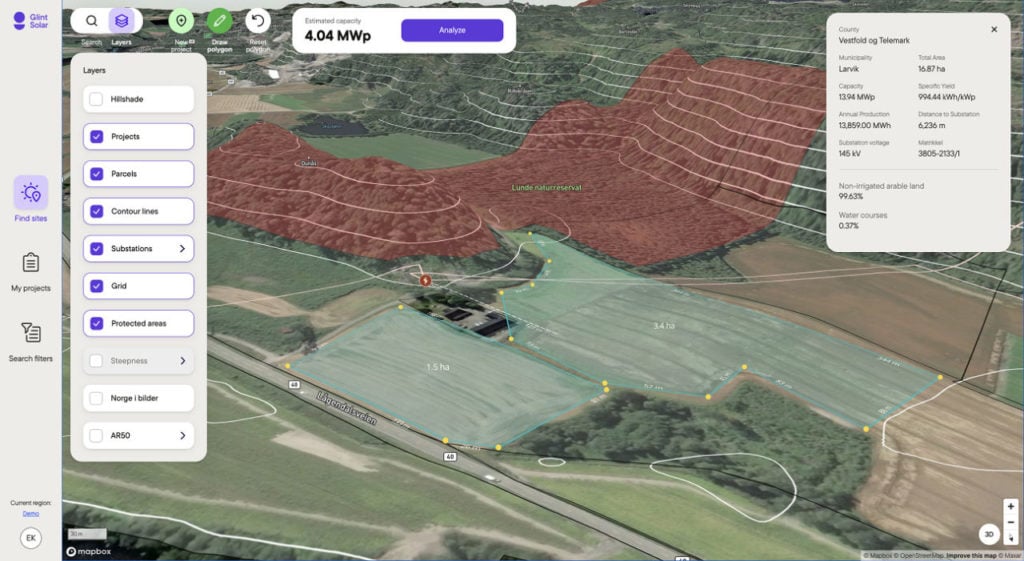
In recent years, the potential for artificial intelligence (AI) and other advanced software technologies for the rollout of solar power has started to be realised. As the scale of the sites has grown, so too has the importance of their placement and design, requiring more elements to be considered than ever before.
“To us it’s surprising how many very sophisticated solar developers are still using the old way of sourcing land: reactively waiting for someone to recommend a piece of land or guessing by looking at Google Earth,” says Even J. Kvelland, co-founder and chief operating officer of solar AI company Glint Solar.
Unlock unlimited access for 12 whole months of distinctive global analysis
Photovoltaics International is now included.
- Regular insight and analysis of the industry’s biggest developments
- In-depth interviews with the industry’s leading figures
- Unlimited digital access to the PV Tech Power journal catalogue
- Unlimited digital access to the Photovoltaics International journal catalogue
- Access to more than 1,000 technical papers
- Discounts on Solar Media’s portfolio of events, in-person and virtual
“Given how ambitious plans virtually all developers have, they increasingly must be proactive about site screening and we’re proud to finally offer them a software for this important task.”
So how is AI stepping up to ease not just site selection, but design and development?
Software to streamline solar site selection
Advances in software are allowing developers to assess a vast range of elements faster than ever, from the very early stages of site selection right through the development.
According to the Solar Energy Industries Association, depending on the specific technology, a utility-scale solar power plant can require between five and ten acres per megawatt of generating technology. The land this is then developed on will generally require some grading of land and clearing for vegetation. Solar PV can be built on land with a slope and no water access, but there are considerations around shading and topology amongst others.
With solar farms now being developed by the tens of megawatts, this is often a huge amount of land that needs to be assessed.
“We’ve built a very intuitive yet powerful software-as-a-service (SaaS) platform where the solar developer themselves identify and analyse potential project sites,” explains Kvelland. “They can for instance identify specific types of land, such as industrial zones or pasture or identify relevant parcels where you can for example build min. 10MWp and is no more than 3km from a grid interconnection point. From there they can analyse topography and run more in-depth technical and financial analyses. In sum, we are streamlining the early screening work to quicker build a robust project pipeline.”
Glint’s software for example allows solar developers to explore a number of elements of site selection, but Kvelland says the most common are a combination of irradiation, distance to interconnection, topography, and environmental constraints.
“We are attempting to pull in as much relevant insight in the early planning stages as possible to give our customers – the solar developers – the best possible foundation for optimising their pipelines,” continues Kvelland.
“Knowing which projects to kill and which to go for is tremendously important from an efficiency point of view. AI can be used to detect what type of land satellite imagery is showing, if a waterbody for floating PV is natural or man-made and we have been working on an AI model to assess the bathymetry of lakes from satellite images based on how much light is reflected from the bottom.”
On a residential scale too, AI is stepping in to help provide accurate and rapid estimates for the solar potential of homes. Numerous software programs are able to offer installation designs that demonstrate how to maximise the roof space available to generate the most power.
Oil and gas giant TotalEnergies and Google Cloud for example launched an AI tool dubbed Solar Mapper to help accelerate the deployment of residential solar panels in 2020.
Using AI to ease grid connections
It is no secret that one of the biggest challenges for the rollout of clean energy technologies like solar is network capacity. In the US, a Lawrence Berkeley National Laboratory analysis in April 2022 found that there was almost 1TW of renewable energy capacity – of which 700GW was solar PV – in interconnection queues as of the end of 2021.
A similar story is true in other markets, with long connection times in countries like the UK and Spain for example. Here too, AI is stepping up to help tackle challenges around grid capacity however. “There are a number of ways in which AI can really benefit the further development of solar,” says Kvelland. “In the early stages, one can apply various AI algorithms to do more advanced assessments of capex and opex with regards to choosing specific sites and the plant design at those sites. One can run prediction algorithms on future power prices and where to build and how to feed into the grid in combination with storage.”
Grid connection challenges impact the rollout of solar power at all levels, from the largest mega-projects to individual residential connections. One project looking to use AI to improve grid connections in the US is being run by a team at Stanford University, who have developed an algorithm that allows them to map residential solar across the country, and in doing so, understand its impact on networks.
Using satellite imagery from Google, the team – led by Ram Rajagopal, associate professor of Civil and Environmental Engineering at Stanford University – has been able to track the widespread adoption of both rooftop PV systems and grid-scale solar, digging into what change is needed from system operators, utilities and solar system providers to manage and plan ahead for new deployments.
“One of the main issues [utilities are] having is they need to do the planning for the short, medium term and long term,” says Rajagopal.
“And one of their big concerns is what if there’s too much solar behind a particular transformer or which region solar is going to come in. So when they do their ten-year plans, they take those things as inputs, and they really like the power adoption curves. Because solar is very concentrated in certain locations, and as costs come down and incentives change, suddenly locations are having onset. When you have an onset, your previous data is not really a good predictor of what’s going to happen in the future.”
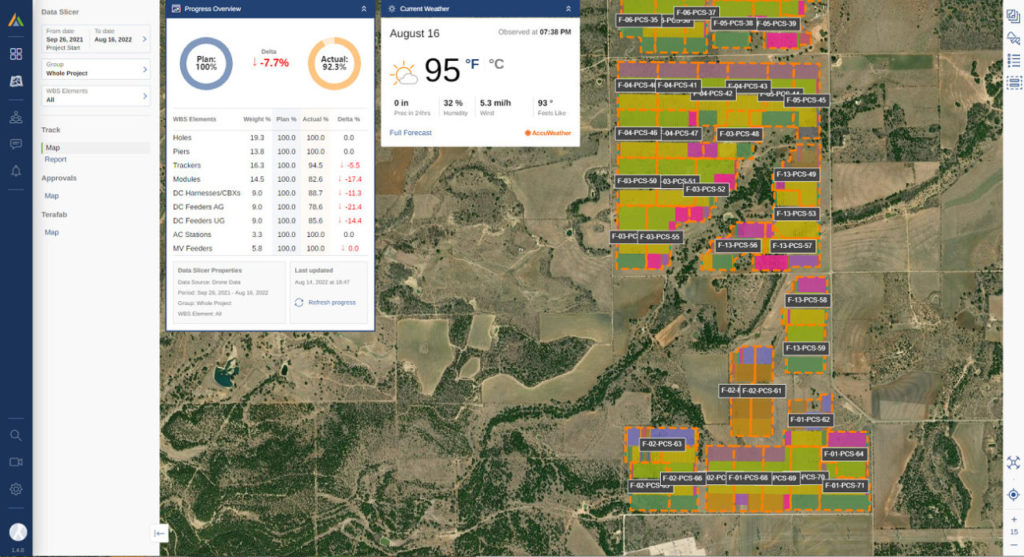
The sophisticated software easing construction
Once a site is selected, taking into account all major inputs such as the quality of the land and the availability of a grid connection, software is also stepping up to help with the construction of solar sites as well, with the process becoming increasingly automated to maximise efficiencies.
One company that is looking to employ advanced software in the next stages of solar site development is TeraBase. Born out of a digitalisation programme at SunPower, the company looks to use automation and digitalisation to ease the rollout of utility-scale solar power.
“The first thing we do is we create a digital twin of the plant,” explains Matt Campbell, CEO of TeraBase, which then acts as a full, 3D plan for the site.
“We have a pop-up factory where we bring in steel structures, cables, panels, and then we use a combination of robotics and manufacturing machines to assemble everything. So we build these structures that are about 13-metres long, and then have a special vehicle, which will be autonomous – it’s not autonomous today, but it will be – and that delivers the structures to the field and installs them. So we have a fleet of vehicles, and they’re just in constant operation, almost like ants just following the path.”
The company has now completed its first beta project and is starting to unpick the benefits of automation and digitalisation for the construction of solar power farms.
This includes numerous health and safety benefits, as well as efficiency of rollout and environmental improvements. The company’s beta project is in central Texas where developers are contending with 38°C heat, rattlesnakes and dust storms, making health and safety a particularly key consideration. By automating the construction, workers could minimise the time spent outside at the mercy of such elements, as well as avoiding “lifting these 40kg panels all day”.
Intelligent optimisation once operational
Once the site is selected and developed, AI and other advanced software can also come into play to optimise the operation of the assets. Back in 2019, a project from National Grid ESO in the UK found AI could improve its solar forecasting by 33%.
Other initiatives are emerging to explore the use of AI for optimisation of solar sites, with Kvelland pointing to collaboration between IBM and the US Department of Energy, which “looked into self-learning weather models, sensors and satellite imagery and was able to achieve 30% better solar forecasting”.
“AI will also be very important not just on the supply side, but also on the demand side and how to balance the two. Once built, one can use AI to improve cleaning and maintenance, which is especially very attractive on large PV plants.”
From cradle to grave, AI and other advanced software platforms are easing the rollout and operation of solar power. Whilst there are still undoubtedly challenges around the availability of data, the integration of processes and the development of the hardware to match it, AI looks set to play a key role in solar design going forwards.