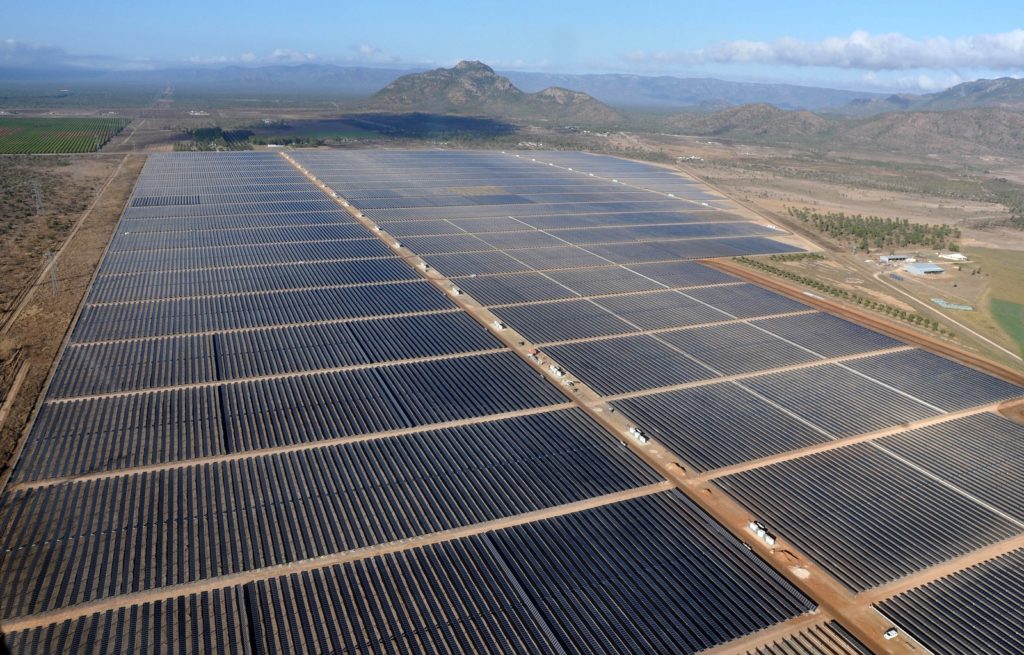
Accurate generation modelling and forecasting is integral to not just the financial performance of operational solar farms, but how they are integrated into modern grids. A study from Monash University in Australia has proven how artificial intelligence approaches can help drastically improve performance forecasts. Dr. Christoph Bergmeir, who led that study, details its key learnings.
Predicting short-term energy generation is not an easy task. Renewable energy cannot be produced on demand, as it is bound to natural resources such as the wind and sun. Therefore, to achieve a stable network and enough power generation, we need a reliable short-term prediction method. In a cross-collaborative research project led by myself from the side of Monash University, Australia, I worked with Worley and Palisade Investment Partners to carry out the necessary trials to solidify more accurate ‘five-minute ahead’ self-forecasting tools.
Unlock unlimited access for 12 whole months of distinctive global analysis
Photovoltaics International is now included.
- Regular insight and analysis of the industry’s biggest developments
- In-depth interviews with the industry’s leading figures
- Unlimited digital access to the PV Tech Power journal catalogue
- Unlimited digital access to the Photovoltaics International journal catalogue
- Access to more than 1,000 technical papers
- Discounts on Solar Media’s portfolio of events, in-person and virtual
The project, which began back in October 2018, was funded by the Australian Renewable Energy Association (ARENA) with a budget of almost AU$1 million. The importance of this project cannot be understated, as by improving the accuracy of five-minute ahead forecasts required by the national electricity market (NEM), the generation forecasting solutions developed by the Worley and Monash team can enable a more secure and reliable grid.
The forecasting models developed are based on machine learning algorithms drawing on internal supervisory control and data acquisition (SCADA) data feeds from the generators as inputs to the model. The 130.8 MW Waterloo Wind Farm (WWF) in South Australia and the 116MW Ross River Solar Farm (RRSF) in Queensland were chosen for the study. The key benefits of the project include increased renewable energy penetration in the grid due to improved dispatchability of renewable generation and reduction in Frequency Control Ancillary Services (FCAS) payments by generators resulting from the failure to meet forecast targets.
A history of inaccurate predictions
The challenge that my team and I were presented with spawned from a history of inaccurate power predictions, which in 2020 alone cost Australian generators AU$210 million. This stems from the fact that natural weather variations can make it difficult for renewable generators to accurately forecast their short-term power generation levels, thus affecting grid stability. Moreover, using machine learning algorithms to see as little as five minutes into the future is extremely valuable.
Our forecasting algorithms attained a 45% improvement in our partners’ power output predictions. This technology has the potential to lower energy prices across the board, and possibly open avenues for other forms of clean energy. If renewable generators can lower their causer pays factors, they can produce electricity cheaper, and eventually that saving could be passed on to the customers. It would also make renewables more competitive.
The machine learning algorithms for both farms are setup to run as an ensemble and deal with the challenges associated with missing data when there are AEMO (Australian Energy Market Operator) imposed curtailment constraints on generation output. During normal operation the model runs using 30 minutes of past historical SCADA data and active power data. When constraints are active, past values of active power are not available to produce a forecast, so the algorithm utilises lags of possible power and other SCADA variables. The third scenario occurs when the generator is coming out of a constraint, but a full 30 minutes of unconstrained history is not yet available. In this instance the algorithm utilises a model with a smaller historical window that also considers ramping immediately after a constraint is lifted. Finally, each model is independently optimised for Mean Absolute Error (MAE) and Root Mean Square Error (RMSE) with the algorithm combining the two together. The combination of these different models running in parallel means that our algorithm can respond more accurately when constraints are active and lifted by switching between models.
Whilst the AEMO self-forecast is theoretically described as a five-minute ahead forecast, it should more accurately be described as a six-minute and 10-second ahead forecast, as the forecasts must be submitted 70 seconds before the five-minute gate closure time. The forecast horizon also needs to consider the delays associated with receiving the data, processing the model and communicating with the AEMO Application Programming Interface (API); approximately 10 seconds. Since minutely data are used, the models were trained to forecast six minutes and seven minutes into the future, then interpolate the forecasts to obtain a six-minute and 20-second ahead forecast.
Each of the forecasting models has been designed to run at the edge on a Dell Edge 5100 Gateway. The edge gateways are combined with the onsite SCADA systems to feed live streaming data over Modbus protocol into the machine learning models. The Edge devices provide local buffering of data and by not transmitting large volumes of live data, the bandwidth needs for internet communication are reduced. It also means the models will continue to run if internet connection is interrupted and stops for any reason, providing a seamless recovery once communications are re-established. Connection to the AEMO API, remote monitoring of model performance as well as patches and updates to the model and edge firmware are all enabled over internet connection.
Inside the results
When looking at the results of the Waterloo Wind Farm performance, 16,123 five-minute dispatch intervals were evaluated over the assessment period. Out of those, 1,442 were constrained and 14,681 were unconstrained. During the valuation period, our algorithm successfully outperformed the benchmark AWEFS (ANEMOS) forecasts. Moreover, our algorithm showed an average improvement of 19.8% on MAE and 45.6% on RMSE during unconstrained periods.
Moving onto the performance of the Ross River Solar Farm, 10,423 five-minute dispatch intervals were evaluated over the valuation period. Out of those, 259 were constrained and 10,164 were unconstrained. During the assessment period, our algorithm successfully outperformed the benchmark ASEFS (ANEMOS) forecasts. Our algorithm showed an average enhancement of 2% on MAE and 8% on RMSE during unconstrained periods.
Whilst numbers and statistics help us define the success of these trials and our research, it is wise to look at the financial benefits of the five-minute ahead forecasting method, as this is where we can provide real value to businesses and consumers alike. As both RRSF and WWF have existing self-service forecasting providers and do not use the ANEMOS forecast algorithms, it was not possible to benchmark the commercial benefits of our self-forecasting algorithm versus ANEMOS at these sites.
To work out the commercial benefits, historical regulation Frequency Control Ancillary Services (FCAS) charges are divided by total generation capacity to determine an approximate cost per MW/year. Regulation FCAS has historically exhibited extreme volatility. Based on this approach, annualised savings are estimated between AU$45,000 to AU$100,000 per year for the wind power forecasting algorithm, and AU$5,000 to AU$20,000 for the solar power forecasting algorithm under ‘normal’ FCAS price ranges. Savings could easily surpass these estimates, particularly during extreme pricing events such as the South Australia separation incident in late January 2020 where even minor improvements in CPF would yield disproportionately large savings.
“Savings could easily surpass these estimates, particularly during extreme pricing events such as the South Australia separation incident in late January 2020”
One of the features of our wind forecasting algorithm is that it is far less prone to over prediction (Kurtosis and Skewness) than the ANEMOS forecasts. High Kurtosis is a measure of the weight of the tails in the error distribution. The high Kurtosis for the ANEMOS forecast means that it is more likely to deliver high forecasting errors than our self-forecasting model. The Skewness of the distribution shows the bias of the distribution towards over or under prediction.
Our model is neutrally biased with a Skewness very close to zero. The ANEMOS forecast is heavily negatively biased, meaning that it is more likely to over predict the generation output than underpredict. The benefits of this behaviour are significant but difficult to estimate. Because the Causer Pays Factors (CPF) calculation sums the deviations over the dispatch period, extreme outliers will inflate the net deviation calculation and therefore attract a higher CPF. As a result, the actual benefits to wind farms may be meaningfully underestimated.
Who benefits?
The benefits of our research and forecasting methods to grids, the market, and consumers are plentiful. The objective of regulation frequency control is to achieve generation/demand balance in response to minor deviations in load or generation. Imbalance results in frequency rises or falls which, if not addressed, can compromise overall stability of the system. The FCAS market is the mechanism that AEMO uses to alter the generation and load balance to maintain frequency stability. With the introduction of self-forecasting, semi-scheduled generators can set their own five-minute dispatch targets.
The forecasting algorithms established for this project have demonstrated an ability to out-perform the AEMO AWEF and ASEF forecasting models which would result in a lower CPF for individual generators. If these improvements were extrapolated across all solar and wind farms in the NEM, the cost of regulating FCAS services across the market as whole would reduce, making renewable energy projects more attractive to investors and driving down the cost of energy for consumers.
The results from this project have demonstrated that improvements can be made to forecasting accuracy for both wind and solar generators by employing best practice machine learning techniques. The results from the wind farm were particularly positive, with significant improvements in both RMSE and MAE. The wind model also displayed a robust ability to maintain accurate predictions even during ramping events, such as after constraints were lifted. The objective of this project was to explore methods for self-forecasting that could develop a more accurate 5-minute ahead forecast, which has been achieved.
Author
Christoph Bergmeir
Dr. Christoph Bergmeir is a senior lecturer in Data Science and Artificial Intelligence, and a 2019 ARC DECRA Fellow in the Department of Data Science and Artificial Intelligence at Monash University, Australia. His fellowship is on the development of “efficient and effective analytics for real-world time series forecasting”. He works as a Data Scientist in a variety of projects with external partners in diverse sectors, such as in healthcare or infrastructure maintenance.