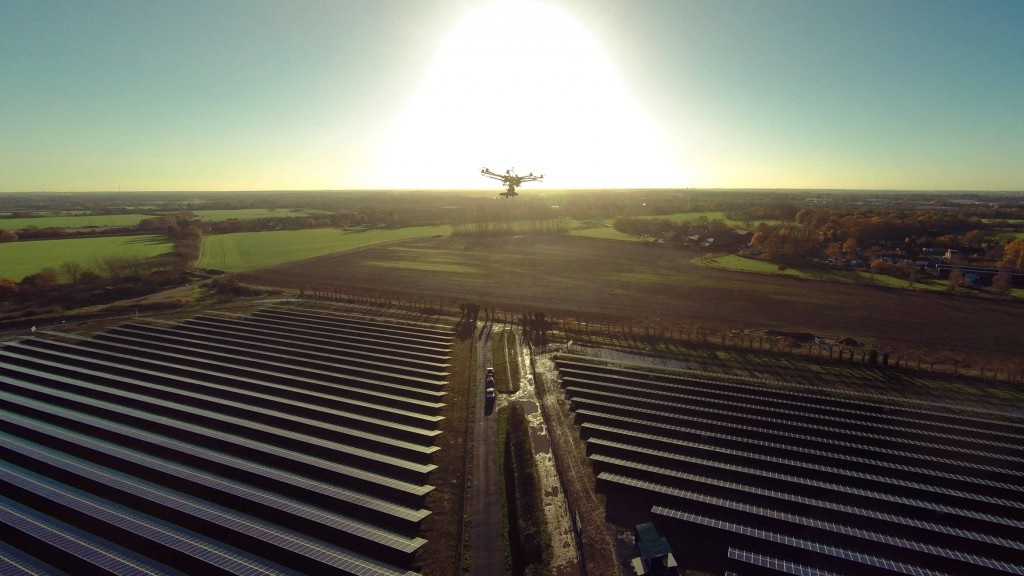
The use of artificial intelligence (AI) is growing in almost every area of business, from banking to transport to healthcare, as the true potential of the technology becomes increasingly recognised. Solar is no different. Within our industry, AI has the potential to transform the way solar projects are operated and managed. When aggregated, enormous datasets from thousands of solar farms can help predict output by analysing trends in cloud cover, radiance and more, while more sophisticated imaging and assessment techniques are improving our understanding of module performance and degradation over time.
Although still nascent in the solar space, AI approaches will almost certainly change the industry as we know it. Those who embrace the technology early may well gain a competitive advantage. PV Tech spoke to two experts in the AI-solar space to examine how it is currently being used and the benefits it can bring.
Unlock unlimited access for 12 whole months of distinctive global analysis
Photovoltaics International is now included.
- Regular insight and analysis of the industry’s biggest developments
- In-depth interviews with the industry’s leading figures
- Unlimited digital access to the PV Tech Power journal catalogue
- Unlimited digital access to the Photovoltaics International journal catalogue
- Access to more than 1,000 technical papers
- Discounts on Solar Media’s portfolio of events, in-person and virtual
Or continue reading this article for free
To actually pin down the term AI is in itself a challenge. Its ubiquity means it has become somewhat of a catch-all term, with any sophisticated software often dubbed ‘AI’. In the context of solar, however, AI should be viewed as software solutions that use advanced analytics and machine learning to help grid operators and asset owners to extract additional insights from datasets and integrate these into their operations. That is according to Hannah Davis, a researcher at Guidehouse Insights, a market intelligence firm that has investigated the use of AI across several energy industry areas.
For Roger French, director of the Solar Durability and Lifetime Extension Research Centre at Case Western Reserve University in Cleveland, Ohio, AI in solar is about using “crowd sourced insights into power plants, ensuring asset owners and operators are better informed about their performance through autonomous learning that considers not only the temporal aspect of solar power generations but also its spatiality”.
Evaluating module maintenance and degradation
Experts agreed that the main way that AI can benefit the solar industry is through its application on solar farms to evaluate when module maintenance is needed, as well as the performance loss rate (PLR) of modules within the plant.
By analysing the output of individual modules within a project, AI can pinpoint which modules are running below their anticipated levels, alerting asset owners or operators as to when maintenance is needed. “AI can be used for understanding and predicting inefficiencies or defects within solar technology,” said Davis.
She gives the example of the use of drone technology coupled with AI to deliver aerial images of solar parks that, through advanced analytics software, can predict maintenance issues in modules. “AI can be partnered with drones to take high level pictures of solar fields and then use artificial vision to understand where a defect might be in a solar panel”. This not only saves significant expense, considering the manpower it would take to evaluate each module in a project, but also time as AI systems would perform these analyses in near real-time. Already today in the energy industry, unmanned aerial systems and drones for critical energy infrastructure was estimated to be a US$1.4 billion market in 2021, according to Guidehouse.
French and his team started a project this week that has already collected data from thousands of solar PV plants and components from more than 24 companies – including SunPower, Canadian Solar, C2 Energy, SolarEdge and Brookfield Renewables – over four years. The data will be used to assess PLR across the companies’ plants, which, crucially, use modules from a variety of manufacturers.
“PLR is the annual drop in the power output from modules. Over the warranty period, it shouldn’t go down below 80%,” explained French. He said asset owners can use AI alongside vast datasets like his to determine modules of the best quality for their site, as well as identifying defected and inefficient modules which may have been damaged in a much more efficient way.
“If power plant owners have streaming data coming to them from their plants, they can diagnose with greater accuracy and reliability what’s going on,” said French. “Degradation is happening, but why is it happening? They could find shading problems that are causing inefficient modules from obstructions that they can’t see.”
French’s project, when completed in 2024, will become an open-source resource free to anyone. But before that point, the insights gleaned from the data will be shared with the companies involved, which will allow them to improve their operations, said French.
Measuring plant performance
AI will also have a profound effect on measuring plant performance in the future, both French and Davis said. AI systems will be able to analyse weather forecasting data, historical generation profiles, seasonal output levels and expected demand quantities in real time to present a far more accurate picture to asset owners about their energy output and the price they could get for it than is currently possible. “It will tell asset operators how much output they will need to generate without having to over produce,” Davis said.
Through an analysis of 316 solar PV power plants in the US over two years, French has been better able to understand the individual generation levels of plants by using data collated from the entire cohort. The method has been able to measure energy generation with 8% more accuracy, which, when done on scale, improves the understanding of vast amounts of energy generation.
“By looking at the power output of nearby power plants, we can see how bright the sun is and where the clouds are coming from. So, we’re using these nearby power plants to help us be better informed about the performance of every one of our power plants,” he explained.
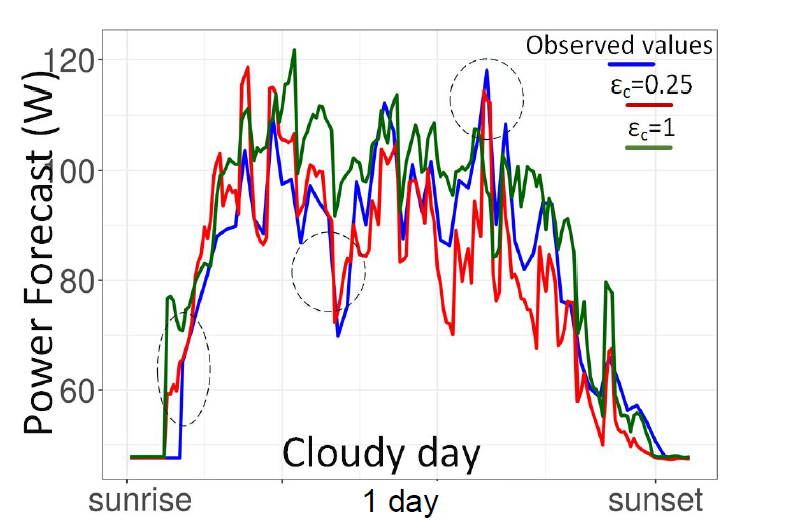
Through a focus on the location of different projects and not just the times of the day when they perform the best, French has been using AI to better predict energy generation of hundreds of powerplants across the US through a model he calls the ‘spatiotemporal graph neural network’. Similar projects in his research centre have been able to predict with 50% more accuracy the power output of certain plants compared to traditional methods.
Asset owners have a few options if they are interested in exploring the benefits of AI for their projects, said Davis. They could develop their own databases and software, which is very expensive but does not involve sharing their critical data. Another option is to buy the software from companies who maintain the datasets it relies on. Or they could outsource AI operations to service providers, but this comes with regular costs and has issues with scalability depending on the provider’s terms. Nonetheless, the options are there for companies that want to introduce the technology.
With AI’s use in solar still in the early stages, it is hard to accurately predict its impact on the industry. But, if one thing is for sure, it is that it has the potential to streamline and improve operations in a way that is currently hard to fully comprehend.