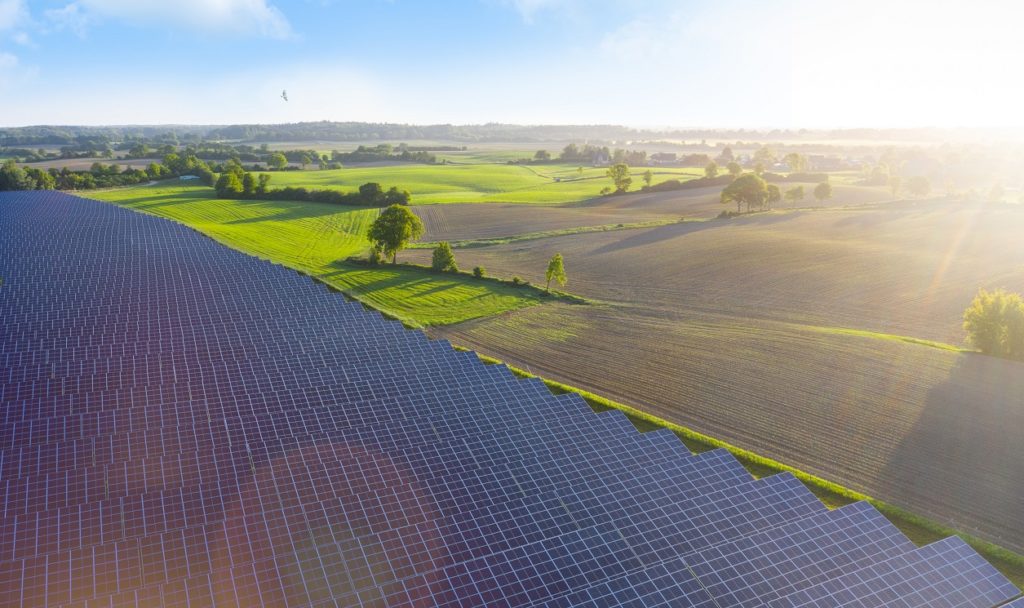
With accurate power forecasting becoming more essential than ever for solar asset owners, Yoojin Lee, a systems engineer and power forecast specialist at Enerparc, reveals how the company’s artificial intelligence approaches are used to make day-ahead forecasts more accurate.
Three years ago, Enerparc started developing a solar PV power forecasting tool as a pilot project in the form of a master thesis from Yoojin Lee, a systems engineer/power forecast specialist at the company. It started as a simple tool for a couple of power plants in Enerparc’s solar PV portfolio and evolved within three years into operational software with a convincing forecasting accuracy.
Unlock unlimited access for 12 whole months of distinctive global analysis
Photovoltaics International is now included.
- Regular insight and analysis of the industry’s biggest developments
- In-depth interviews with the industry’s leading figures
- Unlimited digital access to the PV Tech Power journal catalogue
- Unlimited digital access to the Photovoltaics International journal catalogue
- Access to more than 1,000 technical papers
- Discounts on Solar Media’s portfolio of events, in-person and virtual
In several research papers and publications, the advantage of hybrid models was often addressed to combine two inheritably different models. On this account, the project was designed using a physical model and one machine learning model which was up to date, feasible and appropriate for time series forecasting and combining those two in the end.
The physical model is formed to calculate power output at the grid connection point as accurately as possible, by applying Enerparc’s specific system design details and taking possible power losses into account from PV arrays to the grid. The power output from the physical model is fed into the machine learning model as one of the input parameters.
The adopted machine learning model is called the ‘Long Short-Term Memory (LSTM)’ network as a type of recurrent neural network (RNN), which can model complex multivariate input sequences of observations. The LSTM network has already been very popularly deployed in time series forecasting applications. Meanwhile, many new types of deep learning models have been developed and introduced, therefore it is always important to keep an eye on recent findings and to exchange with people in the field to stay up to date.
AI approaches used by Enerparc
Enerparc harnesses the multivariate LSTM model for time series forecasting. Specifically, multiple variables are put together as input to the model, and only the solar power output variable is predicted as output. The LSTM model is trained based on historical data for each power plant, meaning that Enerparc has 300 different AI models for 300 large-scale solar power plants in Germany. It is decisive to calculate an accurate physical power forecast variable as part of input parameters to run prediction with an AI model. It may sound contradictory to have to calculate physical power to predict a power with AI. The physical power mentioned first is a result of the physical model and is used as one of the input parameters for the AI model. The physical power must be given before being able to harness the AI model. And the final power value from the AI model is the result of prediction after running the trained AI model.
One of the challenges lies in getting physical power as accurate as possible. Enerparc’s solution was to calculate power output values with an internally further developed physical model, based on an academically published power output model. In short, the solar PV power forecasting project harnesses a hybrid model in a combination of a physical model with a multivariate LSTM model.
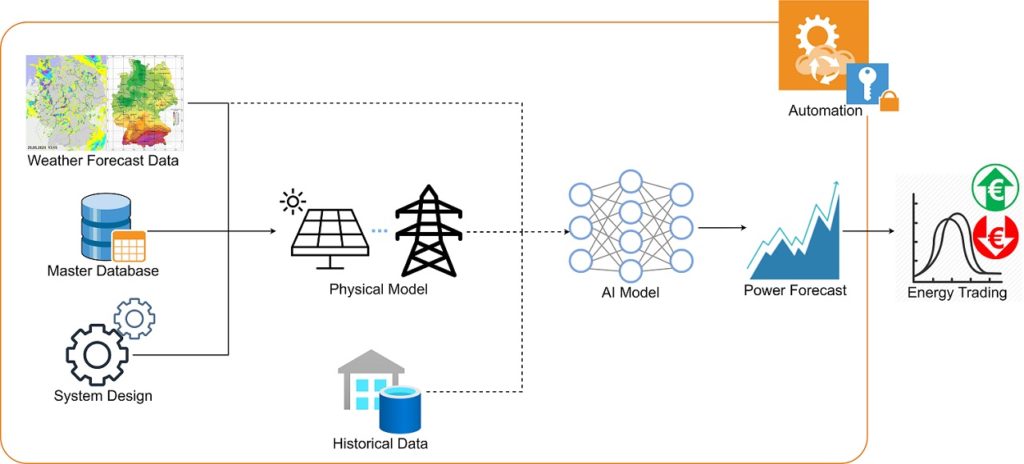
How have they been assimilated into the company’s operations?
Once the prediction performance of the hybrid model was validated, the next step was to establish a sustainable and secure environment of operations with the help of IT experts. There is a long list of tasks to achieve a certain level of reliable operation. For example, a test programming environment should be separated and isolated from an as-built environment, where a tiny change affects the current running operation directly. Logging and notification systems have to be implemented in the case of any possible failure during operations to manage, detect, and analyse issues.
In the beginning, there were many learnings from a variety of errors over time during operations, because building a sustainable environment required specific IT expertise, which was a challenge to learn and implement in a short time. For this reason, it was rather a recurring process of problem-solving with each new error. However, after receiving the appropriate support and expertise from Enerparc’s IT team this power forecast project could finally be running in a reliable, secure and sustainable environment. Accordingly, regular improvements were gradually made to improve the overall operation of the power forecasting model within a couple of years.
Data used for AI
With the forecast of a clear-sky day, it can be easily assumed that a large amount of solar PV energy will be generated. How do humans know the relation between clear sky day and high solar PV energy generation? That is because it has been observed and remembered from the past. For a deep learning model to know and learn the same relation as humans, it needs to learn from the past observations that are being fed into the model. On this account, it is important to feed the right input data into the AI model, which is well-prepared power and weather input variables data in this case.
At first, the input dataset consisted of power generation and a couple of weather parameters. In the meantime, temporal and installed capacity parameters were being added to clarify the nature of data inputs more explicitly, so that each AI model is trained based on more distinct information about each time series data. Especially the installed capacity information was necessary for power plants, which were extended after a while additionally next to the existing power plants. Then the new extended power plant will feed electricity into the same grid point as the previously installed grid point for the existing power plant. The AI model will be able to be trained better with this increasing installed capacity history because it provides a reason why the historical power profile shows bigger values from a certain point.
Importance of central master data
When a portfolio grows over time and a physical model requires more detailed system design information for each power plant, the central master data gets longer and grows exponentially at the same time. Therefore it is absolutely necessary to maintain around-the-clock, up-to-date master data. For example, newly commissioned power plants should be added to the master data as soon as possible, so that power forecasting covers the increased actual portfolio as well. If an installed capacity for a power plant is mistyped in the master data, it will end up overestimating or underestimating the power plant consistently, without knowing why it happens. It makes it even worse in the case of large power plants. That is why it is so important to regularly update and maintain the master data.
Benefits of AI
Power forecasting with AI techniques provides more accurate output in comparison to a single physical model prediction, by learning correlations between input variables and output variables from historical behaviours. There is a clear limitation on the use of a physical model only for time series forecasting. A physical model is calculating the power generation from a mathematical equation with relevant weather forecast parameters. Even if a physical model is formed with numerous variables either in an experimental or theoretical way as accurately as possible, it is incapable of solving an arbitrary complex nonlinear relation between input and output variables. That is why AI, specifically deep learning neural networks, are becoming such powerful and popular tools.
Enerparc’s day-ahead solar PV power forecasting has demonstrated promising and impressive outcomes for its portfolio in comparison to other external power forecasting providers. It led the energy trading team of Sunnic Lighthouse, a subsidiary of Enerparc, to reduce the number of external power forecasting providers from three to two since 2020 by substituting the third-best provider with Enerparc’s forecasting.
Apart from that, it is extremely valuable to own an independent in-house power forecasting product and technology since an accurate power forecasting capability has become more essential than ever before.
Moreover, there are a few dominant power forecasting players in the German market. No matter how big or well-known the companies are, no one is immune from delivering less accurate forecasting results some days or even frequently for some power plants. In other words, it is possible, and it happens from time to time, that every energy trading company overestimates or underestimates power generation by a large margin for the next day, causing massive chaos for all traders, because they all referred to the forecast data from the same well-known forecasting company. However, Enerparc will be less dependent on such a dominant player once the power forecasting technology lies in its hands and delivers an additional reliable reference.
At the moment it is being optimised further regarding the forecasting results by comparing a forecast outcome to the real-time power measurement values, which are accessible from Enerparc’s internal database to increase the forecasting accuracy.
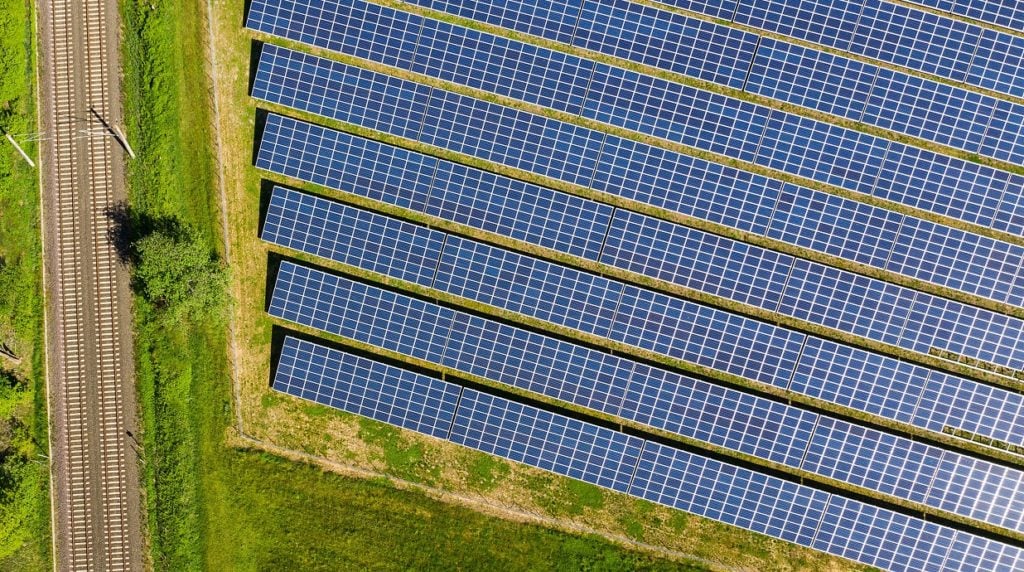
Insights gained from the AI project
Before starting to work on an AI project, three elements are truly essential: good quality of data, an optimised deep learning model and a good team with expertise.
Good quality of data
Data cannot be regarded as separate from any kind of AI project. A good result of an AI project is consequentially preceded by the high quality of input data, which is finely prepared after collection. In the case of power forecasting, there are two main datasets: historical data and weather forecast data. Once historical time series data for desired input variables are collected, the very first step is to identify, clean, filter and handle missing and wrong data. This is one of the most time-consuming tasks but very important to get the most out of the given data. Secondly, weather forecast data is the main source to achieve an accurate power forecast. Many weather forecasting providers are harnessing their machine learning models to optimise weather forecast data from different sources. In Enerparc’s case, weather forecast data is being used from DWD’s (German Weather Service) open data FTP server for its solar PV power plants in Germany.
An optimised deep learning model
Building a deep learning model is one thing and optimising it is another. Even if two different companies apply the same type of deep learning model, it can generate a completely divergent consequence. There are numerous hyperparameters to optimise deep learning models, which characterise each model and determine the quality of prediction capability. For example, the number of hidden layers, the number of neurons for each layer, the type of activation function for the hidden and dense layers, and the learning rate are part of hyperparameters. Random search or grid search can be used for model hyperparameter optimisation, which is available in the scikit-learn Python library. If there are multiple power plants in a portfolio, it is recommended to train a deep learning model for each power plant, rather than to apply a general model for all different plants.
A good team with expertise
The high quality of an AI project for power forecasting can be realised from the synergy of experienced engineers, data scientists and people standing on the border between them. Data scientists play a very decisive role to bring such a project up to a professional level of operation and result in discoveries that haven’t been noticed before. However, when talking about AI projects, it often tends to be highlighted for jobs of data scientists too much, because it is an unfamiliar and little-known area to many people. Any AI project can also be approached purely statistically without knowledge of the data, and it can work out well enough. However, at least in the solar PV power forecasting field, it is very important to work in a team who have a deep understanding of data in context and correlation with other parameters. For example, how solar PV power can be calculated with meteorological variables and technical system design, what kind of weather parameters can influence power production and what could be the cause for a short sudden drop in power generation during the day, and so forth. In addition, a person being able to speak and understand both languages of engineering and data science plays a huge role because this person can be the bridge to connect two different worlds into one together.
Today’s challenges
Is weather forecast data the best among others?
In general, there are many big steps to finally obtain a power forecast result, starting from collecting data to delivering power forecast data to an end user. Since each step is so closely connected, there are always high chances to accumulate errors consecutively. The first step all starts with weather forecast data. Just like really good food is made from high-quality ingredients, good weather forecast data is highly significant for accurate power forecasting. One of today’s challenges is to find out such good weather forecast data. There are various weather forecast models from different sources in the market that allow access to numerical weather prediction models, offering forecast horizons up to several days ahead. Until now, Enerparc has been applying weather forecast data from DWD exclusively for power forecasting. At the same time, current weather forecast data is being verified and alternative models are being searched for, which have the potential to perform better than what has been used so far
Is there any other input data to add to the AI model?
It is a challenging task to form and structure an input dataset for the AI model. Historical power values are the results of many complex dynamic circumstances that have to be interpreted in the right way. It is very important to be aware of all the possible factors, which influence the power value. That is because it is directly related to shaping input data for the AI model to forecast power output.
When a power plant is extended additionally after a certain period or it is partially disconnected from the grid for repair, component replacement, or inspection, or requested not to feed into the grid for a while to maintain grid stability, the AI model can generate and train a better prediction model, if such information is provided as an input data as well. Besides, a weather input variable can also have a meaningful impact on the power output variable, which is not discovered and experimented with so far. Therefore, Enerparc is working on brainstorming and integrating additional input variables to enable AI models to train and predict more accurate power output results.
With the further development of power forecasting, Enerparc is committed to improving the reliability and predictability of renewable energies which play an increasingly important role in a stable power supply with rapidly increasing green power sources.
Author
Yoojin Lee is a systems engineer and power forecast specialist at Hamburg-based Enerparc. She works on the development and operation of a power forecasting project as well as on the BOS analysis, inverter selection and battery design tool development. Born in Seoul in 1991, Yoojin studied a bachelor’s degree in Mechanical Engineering at the Korea Advanced Institute of Science and Technology (KAIST). She then moved to Germany to deepen her knowledge of power plant engineering and electricity generation through a Master of Science in Power Engineering at the Technical University of Munich.