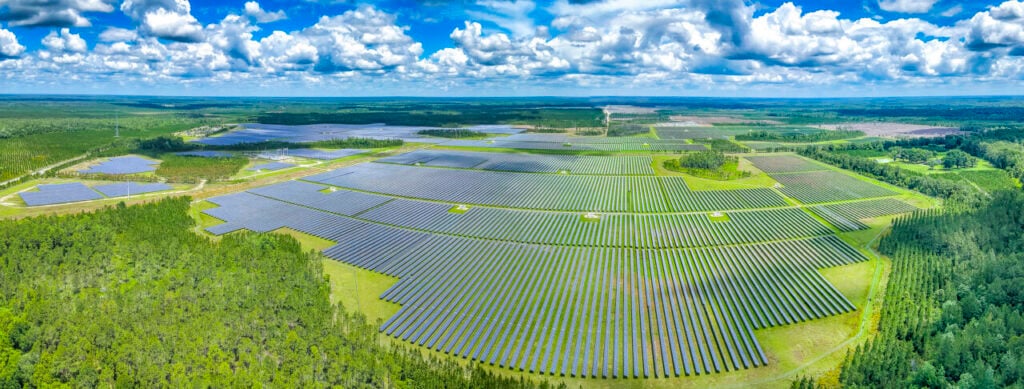
As solar deployment increases around the country, monitoring solar plant performance at a large scale can be quite challenging and interpreting it can be like determining why a string of Christmas lights is out. Sometimes the reason is obvious—the whole string of lights is out, like an inverter malfunctioning in a solar plant. But often, a single light bulb is out, and you cannot figure out why. For solar system operators, understanding whether a setting is causing a performance issue or whether it’s a faulty module, string of modules, or a tracker to blame, can mean wasted time and lost energy production. That is where artificial intelligence (AI) can help.
PV systems need to last for several decades in harsh and changing outdoor conditions. The US Department of Energy (DOE) Solar Energy Technologies Office (SETO) is leading research efforts to understand what causes photovoltaic (PV) systems to fail, how to improve durability, and how to ensure systems last a long time—all key components of reducing the cost of solar energy. One promising application for AI being examined in several DOE projects is analyzing and optimizing the performance of solar systems by reducing human error and identifying common system failures.
Unlock unlimited access for 12 whole months of distinctive global analysis
Photovoltaics International is now included.
- Regular insight and analysis of the industry’s biggest developments
- In-depth interviews with the industry’s leading figures
- Unlimited digital access to the PV Tech Power journal catalogue
- Unlimited digital access to the Photovoltaics International journal catalogue
- Access to more than 1,000 technical papers
- Discounts on Solar Media’s portfolio of events, in-person and virtual
For example, SETO awarded $1.5 million to the University of Maryland, College Park to develop a new approach to assess the reliability of power electronic components in PV inverters. The project team uses machine learning to sort through and interpret 10 years of performance data, making it easier to predict and avoid inverter failures. Without AI, analyzing such a large data set would be prone to errors and challenging at best, or more likely impossible. For instance, a human might sense a change in performance but not be able to correlate such a change with a meaningful problem due to the data’s complexity and size.
Machine learning techniques can help determine when and where to send field technicians for maintenance, ensuring their solar projects run efficiently and maximize electricity generation. By indicating potential problems early, plant managers can minimize downtime and extend the lifetime of PV systems. In 2019 and 2020, DOE funded projects at Electric Power Research Institute (EPRI), Arizona State University, and Stony Brook University to provide real-time detection, classification, and prediction of system failures. Researchers can use data to pinpoint inverter-level issues and predict failures, enabling plant managers to schedule preventive maintenance. In a 2023 DOE workshop that focused on the applications of AI/ML in the solar industry, EPRI and its partners Turbine Logic and Georgia Tech shared their progress and challenges, highlighting the importance of blending machine learning with physics-based models.
Beyond finding the location of a fault, machine learning can also help identify if ageing, weather, or something else caused the failure. A DOE-funded project at the University of Central Florida uses imaging to spot defects across several fields of solar modules. Machine learning techniques help them select modules to bring back to the lab to investigate, helping plant operators understand if flawed manufacturing was to blame for a failure, or if a specific material in the module is degrading.
AI helps researchers compare data across PV systems, finding patterns to streamline maintenance. While current research efforts focus on utility-scale systems, these AI tools could also benefit the residential solar industry. Remote monitoring and automated problem detection could improve performance for third-party rooftop solar owners.
The machine learning models used in PV research are far less energy intensive than the large language models that are driving the growth of data centers and can help maximize the environmental benefits of solar technologies. By increasing the durability and lifespan of PV systems, they reduce waste and keep modules in the field longer. These efforts support DOE’s Photovoltaics End-of-Life Action Plan, which aims to halve recycling costs by 2030 and lower the environmental impact of solar energy modules at end-of-life.
As AI capabilities advance, machine learning could soon support materials development, manufacturing, grid operations and other activities. As the field grows, we are looking for experts to improve and expand datasets, refine analytical techniques, and showcase AI’s benefits to accelerate efficient, resilient, and durable solar deployment.
Lenny Tinker, is Photovoltaics Program Manager at the US Department of Energy Solar Energy Technologies Office.