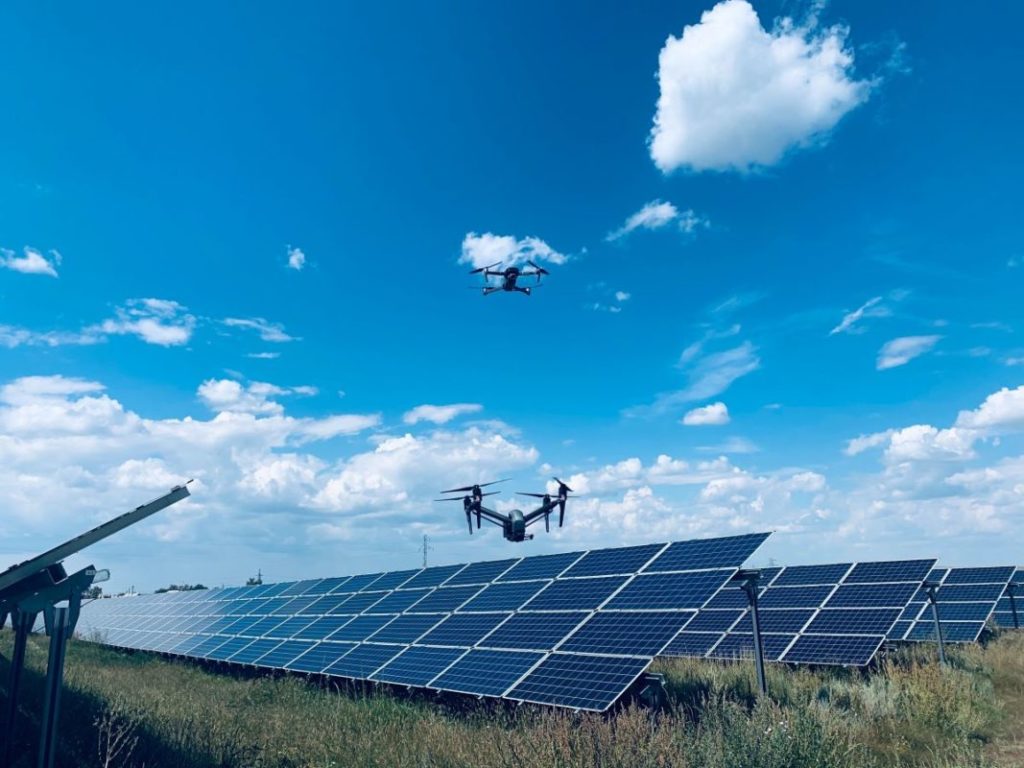
AI-based monitoring systems can help PV plant operators understand and manage the impacts of extreme conditions in a multitude of ways. George Heynes looks at some of the ways AI is being harnessed to bolster system output and longevity.
Unlock unlimited access for 12 whole months of distinctive global analysis
Photovoltaics International is now included.
- Regular insight and analysis of the industry’s biggest developments
- In-depth interviews with the industry’s leading figures
- Unlimited digital access to the PV Tech Power journal catalogue
- Unlimited digital access to the Photovoltaics International journal catalogue
- Access to more than 1,000 technical papers
- Discounts on Solar Media’s portfolio of events, in-person and virtual
Or continue reading this article for free
Across 2023, the globe has been subject to increasingly erratic weather conditions with extreme heat, cold, rain and wind having made their mark. Many of these erratic weather patterns have been attributed to global warming and, with the world forecast to continue its alarming trajectory, this could mean a further increase in extreme weather conditions.
As we have learned in the preceding pages, erratic weather can prove troublesome for solar farms. For example, the efficiency of silicon solar panels drops when an air temperature of 23°C is exceeded. Other extreme weather conditions such as hail and wind can also damage systems thus impacting production yields. With this in mind, it is important to recognise the impact this can have on modelling and how companies are using technology to mitigate the effects of extreme weather conditions – particularly via the use of data and artificial intelligence (AI).
The impact of erratic weather on modelling PV output
According to Neeraj Dasila, chief technology officer and co-founder of SmartHelio, a Switzerland-based software company that has developed algorithms to make solar assets more climate resilient, the impact of erratic weather patterns on PV systems modelling is “profound and multifaceted” with rising temperatures not only reducing the efficiency of the solar module, but also accelerating degradation of critical system components.
Dasila says: “Rising temperatures, for instance, not only diminish the efficiency of solar modules but also accelerate the degradation and damage of critical system components like power electronics of inverters/switches, relays, sensors, etc.
“Additionally, extreme weather events — such as severe storms, hail, and fluctuating precipitation levels — can cause direct physical damage to PV systems, leading to substantial deviations from projected performance metrics.
“This scenario underscores the critical importance of incorporating localised, long-term weather trends into PV system modelling, which is essential for realistic cost-benefit analyses.”
Hail in particular can have a severe physical impact on a solar PV system. Perhaps the most recognisable damage is known as “microcracks”, something that occurs primarily via external impact upon the module. These can have a damaging effect on PV output modelling and thus identifying where the microcrack is located could be paramount.
Will Hitchcock, CEO of smart solar software company Above Surveying, explains that non-visible microcracks can cause issues for a system in the future.
“If the weather is erratic, it can be very difficult to model. If you have a severe weather event, and for solar the main problems are hail and wind, then our services are very well placed to deal with post-event issues and returning the asset to a higher quality state as quickly as possible. It all relies on mapping the damage using drones or other ground-based measurements to chart the change in the modules in different areas. This lets you validate the damage,” Hitchcock says.
This showcases how the use of drones, which incorporate AI technologies, can roam around a solar farm and identify any damaged modules. Conducted on the ground, this can be very time consuming and so it allows damaged sites to return to operation at a much faster rate.
Turning our attention back to the use of data and output modelling, Dasila believes there is a significant lack of “detailed information which hinders the ability of system modelling experts to adequately account for the nonlinear effects of weather and climate change and to tailor their assessments to individual PV installations”.
“The current scarcity of comprehensive studies on the evolving weather patterns and their specific impacts on PV systems exacerbates these challenges,” Dasila explains.
“Consequently, there is an increasing need for advanced analytical tools and methodologies that can effectively manage these complexities and provide reliable, localised insights into PV system performance under changing climatic conditions.”
An abundance of data is a ‘double-edged sword’ for PV
It is common knowledge in the industry that the use of data and AI is core in optimising a solar farm or module. However, Dasila believes this surge in available data can offer a “double-edged sword” for PV yield modelling.
“The surge in available data offers a double-edged sword for PV yield modelling. On one side, it provides a richer pool of information to draw from, enhancing the potential accuracy of models. However, the reliability and relevance of this data are crucial,” Dasila says.
“Inaccurate or irrelevant data can lead to erroneous forecasts, making it imperative for the industry to employ robust data validation and selection processes. We have seen huge deviations, in the range of 10% to 20%, between the projections and the actual performance of the PV installations. Most of these deviations are attributed to poor weather modelling and accurate weather data.”
Despite this, it is worth noting that data can be incredibly useful for creating forecasts for solar PV. For Hitchcock, the use of data forms part of a wider aim to achieve “digitalisation of the asset down to the component”. This can have multiple benefits such as supporting optimisation but, in the context of erratic weather and the damage this can cause, it can help identify issues within a module.
Hitchcock says: “If lots of modules are damaged, then you’ve got a lost yield immediately. But if it’s more subtle, and performance is being impacted by humidity and temperature extremes, we are able to store all forms of testing and inspection data within a digital twin.
This enables patterns in component underperformance arising from weather-related impacts that would otherwise be difficult to spot to be swiftly identified and rectified: “We are able to identify a correlation of, perhaps, a poor performing inverter with low RISO tests on a string of modules. Those RISO tests might be a result of some weather-related impact,” Hitchcock says.
AI in operational decision-making
SmartHelio was brought in by a “major European solar developer” to apply its predictive analytics capabilities to optimise the client’s O&M efforts and minimise overall degradation.
“We used real-time climate and PV system production data along with their historical performance telemetry to develop and train the framework of our predictive models,” Dasila explains.
“For instance, by analysing incoming weather patterns, the AI system pre-emptively predicted the environmental/situational stress on various components of the PV system and predicted their performance and potential failure with an urgent call for immediate action.”
With this regarded as one of the company’s successes in recent years, Dasila was keen to express the useful nature of AI and data, and the potential it could have on the global solar industry as unpredictable climate conditions continue to ramp up.
Dasila says: “[Our] AI model’s capability to forecast potential issues based on environmental factors is a significant advancement in PV system management. The model intelligently predicts the increased likelihood of IGBT (Insulated Gate Bipolar Transistor) failure due to higher operational temperatures, a direct consequence of the heat wave and increased dust deposition. This kind of failure is particularly concerning as IGBTs are crucial for the inverter’s function, converting the DC output of the PV panels into AC for grid integration or use.
“The system’s predictive maintenance capabilities also identify potential component failures, enabling pre-emptive repairs and reducing downtime. This approach has resulted in a marked improvement in both efficiency and reliability, showcasing the transformative potential of AI in managing PV systems under variable climatic conditions.”
Casting an eye to the future for the use of data for yield modelling, Dasila adds: “Future advancements are likely to involve more sophisticated machine learning models that can dynamically adjust to new data, providing more accurate and adaptive forecasting capabilities.
“These developments will also include better integration of disparate data sources, such as satellite imagery and local weather stations, to create a more holistic view of the factors affecting PV performance.”
The role of AI and predictive analytics in PV system operation
As explained earlier in the article, predictive analytics and AI play a huge role in the optimisation of solar assets. By predicting weather patterns and PV output, asset owners are able to detect patterns and predict potential system issues before they occur. In doing so, this maintains the operability of the solar asset and thus increases its output.
This is something Dasila touches on, stating that it is “revolutionising PV system operation, particularly in the face of unpredictable climate conditions”.
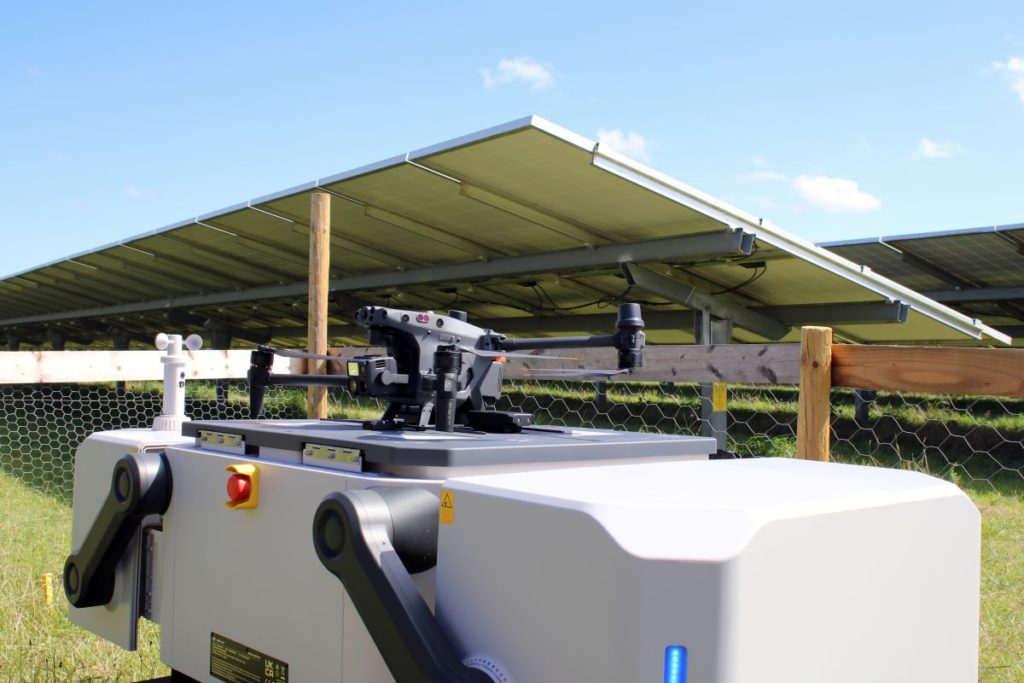
“AI and predictive analytics are revolutionising PV system operation, particularly in the face of unpredictable climate conditions. By harnessing large datasets, AI algorithms can detect patterns and predict potential system issues before they manifest,” Dasila says.
“This proactive approach allows for anticipatory adjustments, reducing the reliance on reactive measures. AI-driven predictive maintenance can also pre-emptively identify components at risk of failure, enabling timely interventions. We bring speed by anticipating a 50% direct reduction in O&M efforts and costs with the use of data-driven advanced analytics and predictive maintenance solutions.”
It is also important to note the impact it could have with unpredictable weather patterns. According to Dasila, the integration of real-time weather monitoring further enhances the solar PV system.
“The integration of AI with real-time weather monitoring systems further enhances this capability, allowing PV systems to dynamically adjust to immediate environmental changes, thereby optimising performance, mitigating risks associated with unpredictable weather patterns and resulting in an increase significant increase in revenues,” Dasila adds.